How to deal with defect detection in continuous or large materials?
A client in the textile manufacturing business, producing yardage and various types of films, needed to detect defects on large-scale materials that deviated from perfect condition.
Images of the production process were collected using a line camera that captures strips of fabric up to 2 meters wide (woven and non-woven textiles). As a result, the images are wide (x-axis) but have a low height (y-axis), leading to unique challenges in defect detection.
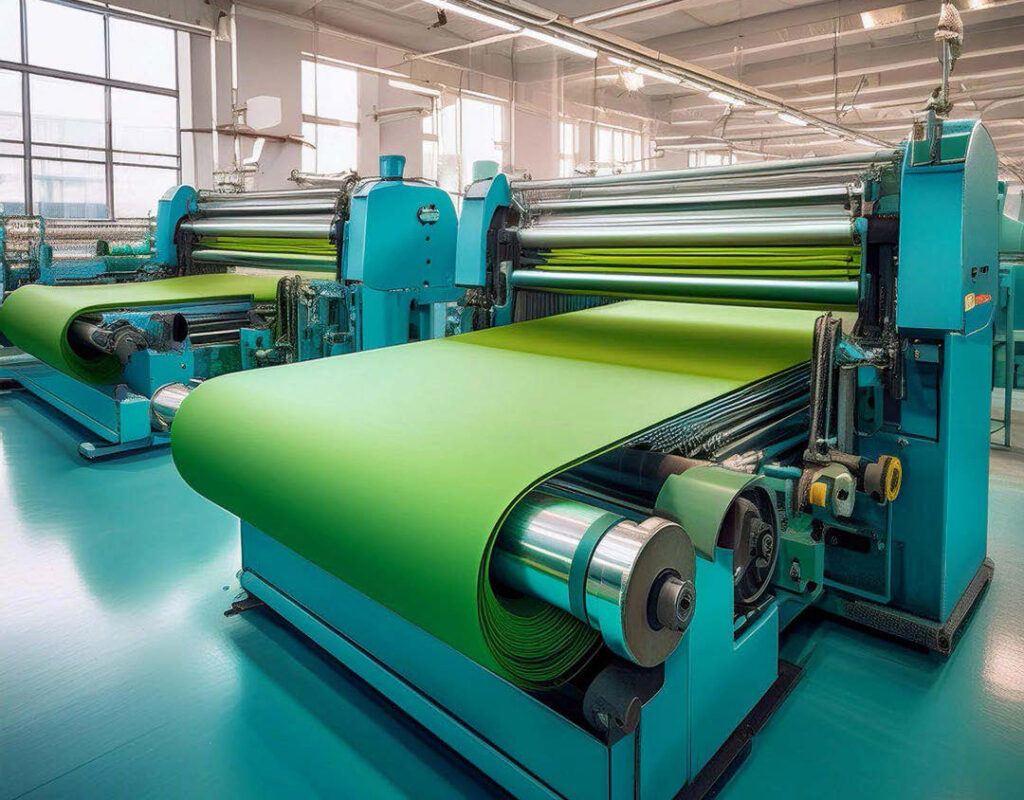
The customer was seeking a simple solution capable of identifying defects of various shapes and sizes—defects that couldn’t be precisely predetermined—at high production speeds. Under normal conditions, this task would typically be handled by a single Anomaly Detector module.
So, where’s the catch?
It turns out that defects sometimes occur exactly at the boundary between two consecutive images. In such cases, the defect could be missed, and the fabric might be evaluated as flawless. To resolve this, we introduced a custom preprocessing step: cropping the bottom 20% of each image and merging that cropped portion with the next image. This overlap ensured that no defects at the boundaries would be overlooked. Instead of evaluating images of 6144 x 420 pixels, we now had 6144 x 500 pixels for each detection—providing extra coverage and accuracy.
Inconsistent Illumination
Another challenge arose due to inconsistent lighting conditions. The conveyor belt was affected by environmental light changes in the manufacturing plant. Depending on the time of day, the weather, or whether the fluorescent lights were on or off, the images appeared darker or lighter. Since the Anomaly Detector is trained on defect-free images and looks for deviations from the norm, these lighting variations could mistakenly be identified as defects.
To address this, we took additional images under varying lighting conditions to properly train the Anomaly Detector module. This ensured that only actual defects were flagged, not just changes in illumination.
Defects Detected—Now What?
When an anomaly is detected in the fabric, the client can classify the load as lower-quality goods and inform the end user of specific imperfections, such as dots, holes, color changes, tears, or structural errors. The end user can then plan their use of the fabric accordingly, making cuts in specific areas to preserve the fabric’s special properties for optimal performance.
This was easy. Got a case that might give us a few wrinkles?
Dealing with anomalies in your production? Contact us for a free feasibility study and let the PEKAT VISION team find the solution for you.